The Association Between New COVID-19 Cases and Google Searches for Mental Health

Table of Contents
Author(s)
Patrick S. Tennant
Former Researcher, Center for Health and Biosciences, Baker InstituteQuianta Moore
Nonresident Fellow in Child Health PolicyJennifer Gonzalez
Meadows Mental Health Policy InstituteMelissa Rowan
Meadows Mental Health Policy InstituteCatie Hilbelink
Meadows Mental Health Policy InstituteShare this Publication
- Download PDF
- Print This Publication
- Cite This Publication Copy Citation
Tennant, Patrick, Jennifer Gonzalez, Melissa Rowan, Catie Hilbelink, and Quianta Moore. “The Association between New COVID-19 Cases and Google Searches for Mental Health.” , June 9, 2020. (https://doi.org/10.31124/advance.12375020.v2).
Tags
The Centers for Disease Control and Prevention projected a substantial increase in COVID-19 mortality (CDC, 2020) across the United States in May 2020. New data suggest that many Americans are feeling distressed by the rising number of COVID-19 cases in their state (Panchal et al., 2020). This collective uncertainty is unlikely to decline given the spread of disease, expanded “shelter in place” orders in many jurisdictions, and the removal of social isolation restrictions in some localities that may lead to repeated waves of infection. The widespread impact of COVID-19 has led to job layoffs or furloughing in a variety of industries, which exacerbates stressors for families across the U.S. (Panchal et al., 2020).
We analyzed Google trends data in an attempt to detect changes in population mental health associated with an increase in COVID-19 cases. Our analyses revealed a positive association between the number of new COVID-19 cases reported and mental health related Google searches. That is, there were more mental health searches on Google in a given state on days when more new cases of COVID-19 were announced in that state. This finding suggests an association between mental health related thoughts (measured through Google searches) and reports of new cases.
Measuring mental health through Google searches is not equivalent to tallying clinical diagnoses, but search trends have been used to capture health care trends (Nuti et al., 2014), population mental health (Soreni et al., 2019), COVID-19 symptoms (Stephens-Davidowitz, 2020) and Zika epidemics (Teng et al., 2017), among other phenomena. Traditional population-based measures often have a multi-year lag time in releasing prevalence and treatment-seeking rates for critical health-related indicators, such as mental illness and substance use (both of which are expected to increase due to COVID-19 (Meadows Mental Health Policy Institute, 2020)). Therefore, high quality measures of the impact of COVID-19 on our mental health may not be fully clear for years. At this point, even a rough measure of population mental health is important to assess so that we can project where the need for mental health services may lie as the pandemic continues. We attempted to remedy this problem using some of the most contemporary data available to demonstrate how the increasing number of COVID-19 cases is associated with population mental health.
We used state-level data from the New York Times COVID Cases database (The New York Times, n.d.) and Google Search Trends (Google Trends, n.d.) to measure the number of new COVID-19 cases per day and Google searches for mental health key words, respectively. Our data represents daily new COVID-19 cases and Google searches at the state level from March 11 to April 11, 2020. We calculated the number of new COVID-19 cases per day (new cases) as the number of total cases reported for each state minus the number of cases reported from the prior day. We then conducted a natural log transformation of new cases because the distribution was highly variable and commonly grew exponentially over time.
Google search trends (obtained from the gtrendsR package (Massicotte & Eddelbuettel, 2019)) were scaled to reflect the relative popularity of a given search term within the state over the queried time period (in this case, 30 days). Values represent a percentage of search volume relative to the highest search volume day, ranging from 100 (i.e., the day with the most searches) to possible low of 0. This score, called the Relative Search Value (or RSV), was calculated for a composite measure called mental health searches, which included independent RSV scores for the search terms “depression”, “anxiety”, “suicide”, and “mental health” in each of the 50 states over the month. The composite of mental health searches was comprised of the average RSV for all search terms on a given state-day.
A multi-level regression model was fit to assess the magnitude of the within-state association between new cases and mental health searches after accounting for date and weekly fluctuations (because mental health searches occur less commonly on weekends). Our results identified a significant association between new cases and mental health searches, such that a 10% increase in the number of new cases in a state would, on average, result in a 0.17-point increase in RSV of mental health searches in that state. There was also meaningful variation in the effect between states (Figure 1 depicts this variation and full model results are provided in the Appendix).
Figure 1 — Mental Health Google Search Trends by New COVID-19 Cases Each Day From March 11 to April 11 for Select States
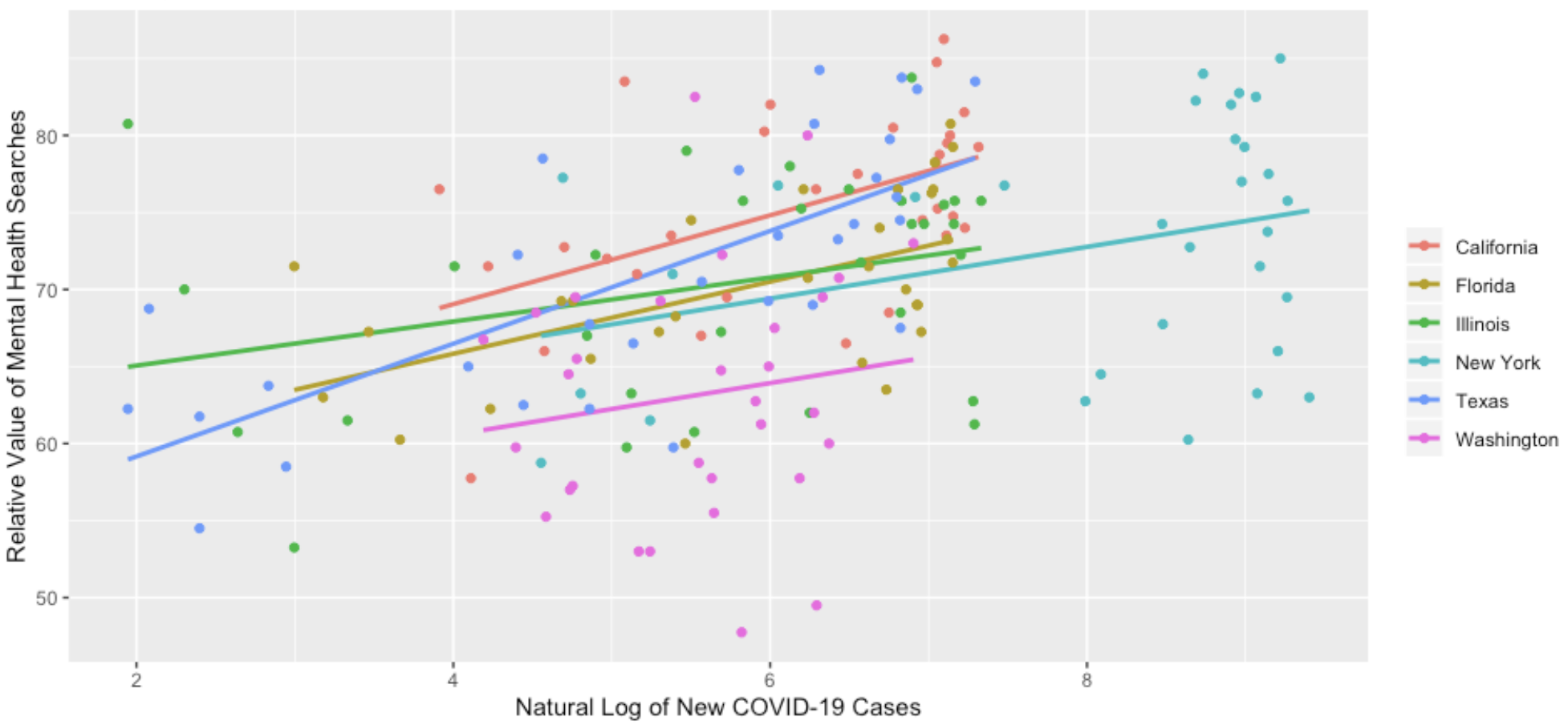
Given patterns of viral transmission and fluctuating testing capacity, our data also include substantial variation in the number of new cases reported on any given day, and thus predicted increases in mental health searches can also be considerable. Fifteen states experienced at least one sampled day with 500% day over day growth in new cases. On those days, our model would predict the RSV for mental health searches to increase by about 3.2 points, meaning potentially thousands more Google searches related to mental health.
We believe these findings could be used by policy makers to encourage utilization of mental health services that are already increasingly available given the federal, state and local responses to COVID-19. Because both state and federal governments have moved quickly to deploy resources, lift restrictions, and change regulatory policy, access to telehealth services has increased. Still, despite reports of increases in demand for tele-mental health services (Robbins, 2020), access to such services is not evenly distributed and many who could benefit do not have access. The people at greatest risk of mental illness—those with a history of adversity, such as trauma or abuse, or who are socioeconomically disadvantaged—may lack the resources (e.g., smart phones with unlimited service plans and cameras) to support telemedicine appointments with a provider. Some steps have been taken to account for the diversity in individual circumstance, but there is more to be done, such as allowing reimbursement for audio-only telehealth services, to continue increasing access, availability, and advertisement of mental health services.
Policy makers should prepare for increased mental health needs in case proposed scenarios of a continued increase of COVID-19 cases, or a resurgence of infections, becomes a reality. That may include continuing to remove regulatory barriers to treatment or insuring adequate reimbursement rates for a variety of levels and types of mental health professional licenses. Though the specific changes needed will vary between states, all levels of government should take steps to make mental health treatment available to everyone as society copes with COVID-19.
References
CDC. (2020, February 11). Coronavirus Disease 2019 (COVID-19). Centers for Disease Control and Prevention. https://www.cdc.gov/coronavirus/2019-ncov/covid-data/forecasting-us.html.
Google Trends. (n.d.). Google Trends. Retrieved April 28, 2020, from https://trends.google.com/trends/?geo=US.
Lorah, J. (2018). Effect size measures for multilevel models: Definition, interpretation, and TIMSS example. Large-Scale Assessments in Education, 6(1), 8. https://doi.org/10.1186/s40536-018-0061-2.
Massicotte, P., & Eddelbuettel, D. (2019). Package ‘gtrendsR.’ https://cran.r-project.org/web/packages/gtrendsR/gtrendsR.pdf.
Meadows Mental Health Policy Institute. (2020). Projected COVID-19 MHSUD Impacts, Volume 1: Effects of COVID-Induced Economic Recession (COVID Recession) (pp. 22–31). https://www.texasstateofmind.org/uploads/whitepapers/COVID-MHSUDImpacts.pdf.
Nuti, S. V., Wayda, B., Ranasinghe, I., Wang, S., Dreyer, R. P., Chen, S. I., & Murugiah, K. (2014). The Use of Google Trends in Health Care Research: A Systematic Review. PLoS ONE, 9(10), e109583. https://doi.org/10.1371/journal.pone.0109583.
Panchal, N., Kamal, R., Orgera, K., Muñana, C., Apr 21, P. C. P., & 2020. (2020, April 21). The Implications of COVID-19 for Mental Health and Substance Use. The Henry J. Kaiser Family Foundation. https://www.kff.org/health-reform/issue-brief/the-implications-of-covid-19-for-mental-health-and-substance-use/.
Robbins, R. (2020, April 13). Will the coronavirus be a breakout moment for virtual mental health care? STAT. https://www.statnews.com/2020/04/13/remote-mental-health-livongo-omada/.
Soreni, N., Cameron, D. H., Streiner, D. L., Rowa, K., & McCabe, R. E. (2019). Seasonality Patterns of Internet Searches on Mental Health: Exploratory Infodemiology Study. JMIR Mental Health, 6(4), e12974. https://doi.org/10.2196/12974.
Stephens-Davidowitz, S. (2020, April 5). Google Searches Can Help Us Find Emerging Covid- 19 Outbreaks. New York Times. https://www.nytimes.com/2020/04/05/opinion/coronavirus-google-searches.html.
Teng, Y., Bi, D., Xie, G., Jin, Y., Huang, Y., Lin, B., An, X., Feng, D., & Tong, Y. (2017). Dynamic Forecasting of Zika Epidemics Using Google Trends. PLOS ONE, 12(1), e0165085. https://doi.org/10.1371/journal.pone.0165085.
The New York Times. (n.d.). Coronavirus in the U.S.: Latest Map and Case Count. New York Times. Retrieved April 28, 2020, from https://www.nytimes.com/interactive/2020/us/coronavirus-us-cases.html.
Appendix
Table 1 — Level 1 Results of Multi-Level Regression Model Examining the Effect of New COVID-19 Cases on Mental Health Searches
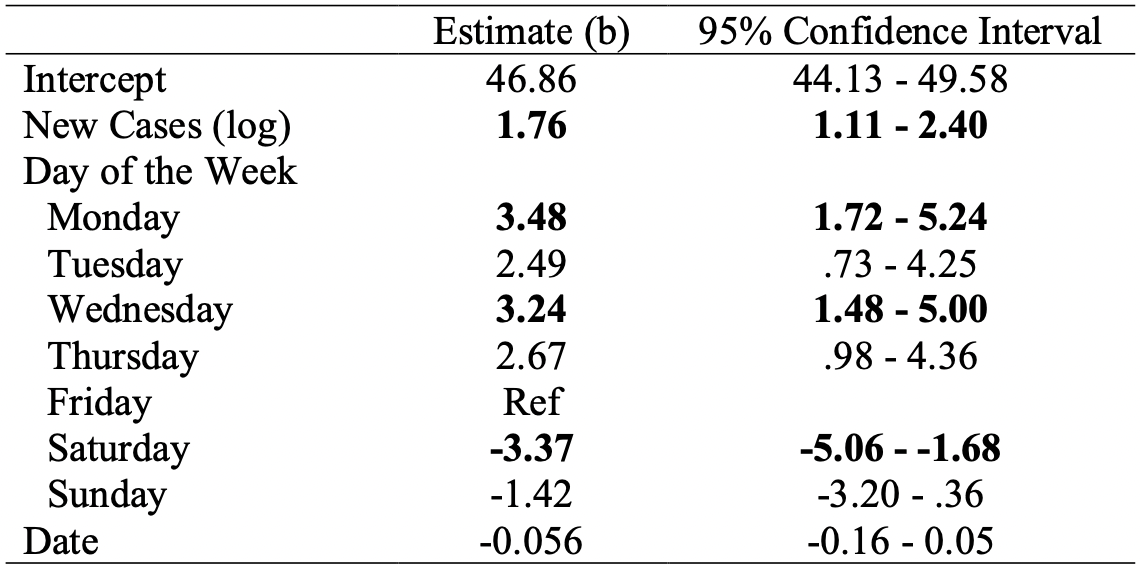
Table 2 — Random Intercepts and Slopes for Each State From Multi-Level Regression Model Examining the Effect of New COVID-19 Cases on Mental Health Searches

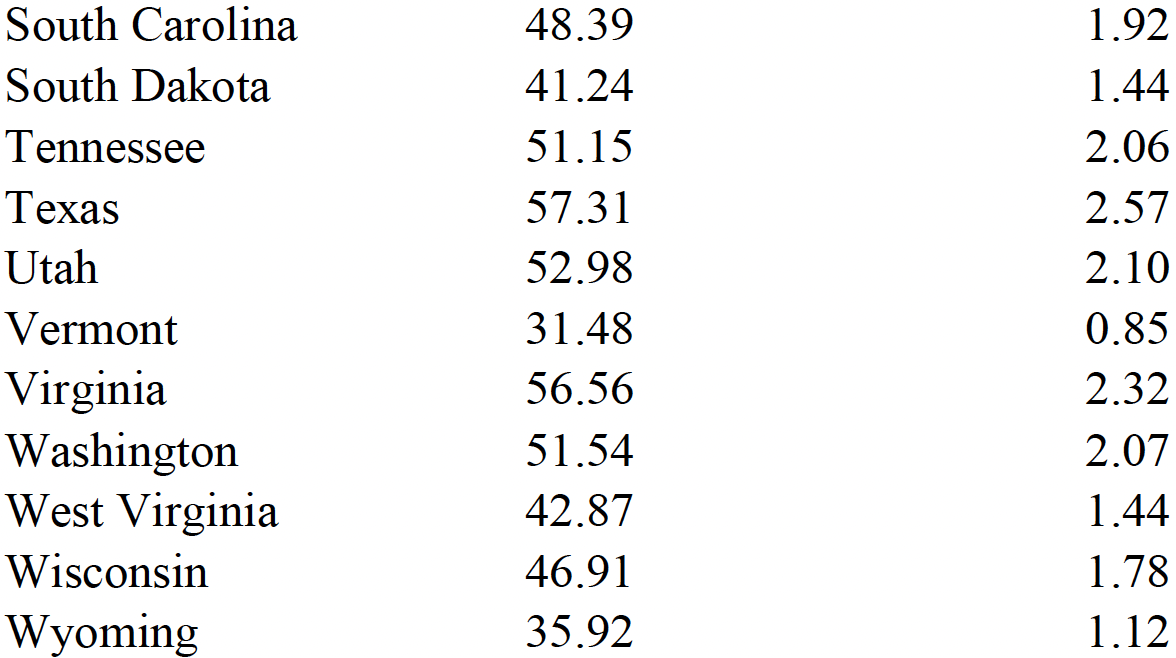
and effect sizes for random slopes is complex and a field-wide consensus
on best practice is lacking (Lorah, 2018), so no measure or indication of
significance is included in Table 2. Still, relative state-level variation in
intercepts and slopes may be of interest to some readers and so intercepts
and coefficients are reported here.