Report on the Effects of Houston-area Red Light Monitoring Cameras

Table of Contents
Author(s)
Matthew Loftis
Graduate Student, Department of Political Science, Rice UniversityAleksander Ksiazkiewicz
Graduate Student, Department of Political Science, Rice UniversityRobert M. Stein
Fellow in Urban PoliticsSummary
This report tracks the impact of red light monitoring cameras on the frequency of traffic accidents in Houston, Texas. We use data collected over seven years to simulate the effects of camera installation and to estimate their impact. The results show that cameras are associated with a long-lasting reduction in the number of collisions at Houston intersections that averages almost 30 percent for single cameras. We find that intersections with one camera benefit from reduced collisions only on the approach with the camera, whereas intersections with two or more cameras see reduced collisions from all approaches. From September 2006 to December 2009, we estimate that camera-controlled intersections in Houston prevented about 792 collisions in total.
Introduction and Description of the Data
This report tracks the effect of red light monitoring cameras on the number of collisions occurring at 50 intersections in Houston, Texas, while dealing with several factors that affect the frequency of traffic accidents. We examine both the direct effect of cameras on preventing collisions and the spillover effect of having multiple cameras at one intersection. The standard procedure in most cities is to camera multiple approaches at each intersection. However, in Houston the practice was to camera a single approach and add additional cameras later. This allowed us to track the effect of adding additional cameras.
Taking into account other factors that affect collisions, we find that both direct and spillover effects of red light monitoring cameras are strong. Interestingly, we only find spillover effects in intersections with two or more cameras. When one camera is installed at an intersection, it reduces collisions from its approach without affecting other approaches. When a second camera is added on another approach in the same intersection, it reduces collisions not only on its approach but on all others as well. The reason for this requires further study, but we will show that the effect is strong and persistent.
Table 1 — Basic Summary Statistics

We acquired collision data by intersection and approach from the Crash Record Information System (CRIS) database1 compiled by the Texas Department of Transportation (TxDOT). CRIS is compiled from paper collision reports from all local public safety agencies in the state of Texas. Public safety agencies are required by law to submit these reports to TxDOT within 10 days of a motor vehicle crash.2 Information was collected between January 2003 and December 2009; the first cameras were installed in September 2006. We filtered the CRIS data to include only collisions occurring within 500 ft. of a DARLEP3 intersection4 in Houston.
Table 1 provides some basic information. Please see Table 3 in the appendix for a full list of intersections and average monthly collisions. Cameras were installed on at least one approaching street in each of 50 intersections by May 2007. An additional 20 cameras were installed in August 2007,5 so a grand total of 70 red light surveillance cameras were installed during our period of study. The data we use was collected on the number of accidents at each of the four “approaches” to these intersections. This makes for a total of 200 individual “intersection approaches.”
How to Measure the Effect of Cameras
Cameras were installed on approaches that experienced more accidents. Between January 2003 and September 2006, before any cameras were installed, the average number of collisions per month was significantly higher on approaches that eventually received a camera than on those that never did.6 This strongly suggests that the approaches that received camera control were not selected at random.
Of course, cameras are not an unlimited resource, so this practice makes sense. However, it does make it complicated to measure what effect cameras have on collisions. It is not enough to simply compare collisions before and after camera installation as we do in Table 1. For example, if high-risk approaches got cameras and low-risk approaches did not get cameras then we can only compare high-risk approaches with cameras to low-risk approaches without cameras. We could compare high-risk approaches before and after camera installation, but this will only help if traffic accidents are basically constant over time. Since there are trends over time, for example due to weather variation or changes in automobile technology, then this is also a problem.
If the Houston program were an experiment, the cameras would have been installed at intersections chosen at random. However, the six years of data we have is the nearest thing to an experiment available. To get around the problems with making simple comparisons, we chose to use all of the data available to us to build a statistical model of traffic accidents. We use this model to simulate the number of collisions at an average intersection approach with or without cameras installed. The simulation approach solves our problem because it provides a baseline for comparison that is built from the information collected at all 50 intersections and 200 intersection approaches and incorporates everything we can measure about what causes traffic accidents.
If our simulated intersection shows a drop in collisions when a camera is installed, then we can conclude with confidence that cameras had a positive effect in Houston. In order to get the best model of collisions, we include information in our model that addresses these key issues:
- Time: Between January 2003 and December 2009, there was a natural decline in the number of accidents across all intersections in Houston. We account for this effect.
- Weather: There are a variety of ways Houston weather affects traffic. We account for the amount of rain each month, the number of rainy days, and the monthly average temperature. We also address the effects of the two major storms during this period: Ike and Rita.
- Geography: The intersections (and their different approaches) we studied are located across the Houston area. Traffic volume, nearness to the freeways, and a variety of other factors make these locations very different from one another and affect the number of monthly collisions.
- Calendar month: Months vary in collision frequency due to seasonal differences in weather and traffic patterns. These include differences from the holiday season, the summer school vacation period, etc.
There are dozens of factors that might affect collisions. Some of them, like weather, we can measure easily. Others, like traffic volume, we do not have measures for. To get around this, we can account for all those unmeasured details indirectly by treating each intersection and each approach as unique.7 Filtering out these unique geographical features, time trends, and seasonal patterns lets us focus in on the effect of cameras, and it also ensures our model is highly accurate. In a test of the model, it correctly predicted all but 25 collisions out of 1,413 collisions between September 2006 and December 2009.
The Baseline for Comparison
Figure 1 — Baseline Prediction for a High-risk Approach; Based on 50 Intersections in the Houston Area, 2003–2009
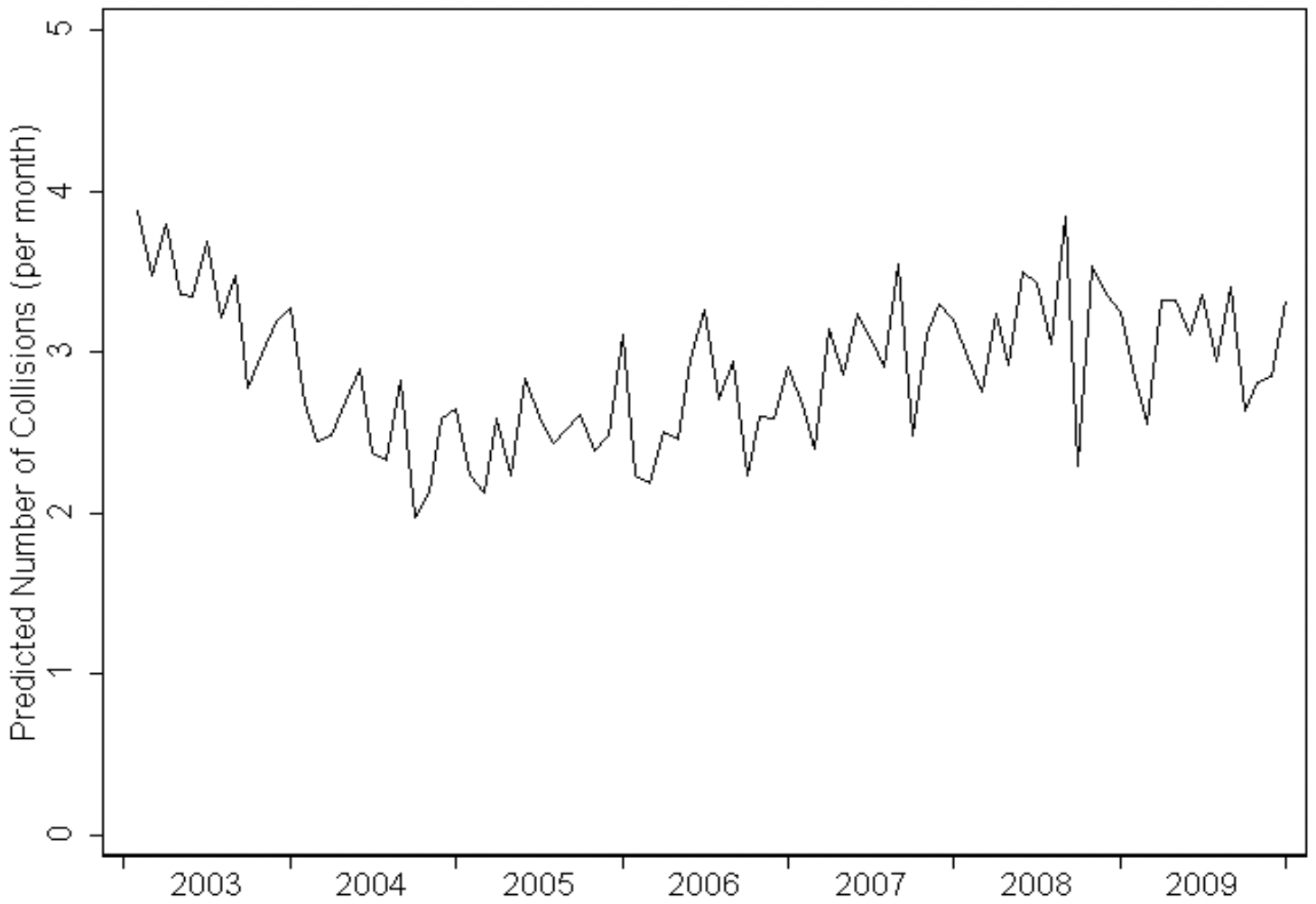
Accounting for all the factors described above, our model8 predicts the baseline number of collisions for an average high-risk approach shown in Figure 1. The area between each set of ticks at the bottom of the graph denotes one calendar year. This is not a plot of actual data, but rather the best prediction from the model as to how many collisions would happen at a simulated high-risk approach each month. Notice that there is a small trend toward fewer monthly collisions as time passes and that the number of collisions varies month to month. This will serve as the basis for comparison in the rest of the report.
The Effect of Camera Installation
Statistical tests showed that camera installation does reduce collisions, but the size of the effect depends on how many cameras are installed, which approach we study, and how long the camera is in place. A single approach with a camera sees a reduction in collisions, but approaches without cameras tend to see reductions only when there is more than one camera installed at the same intersection. In every case, the benefits of having cameras at an intersection grow with time, perhaps as drivers learn to watch for the cameras. The figures below tell this story in more detail.
Figure 2 — Predicted Reduction in Collisions per Month; Camera Installed 09/2006
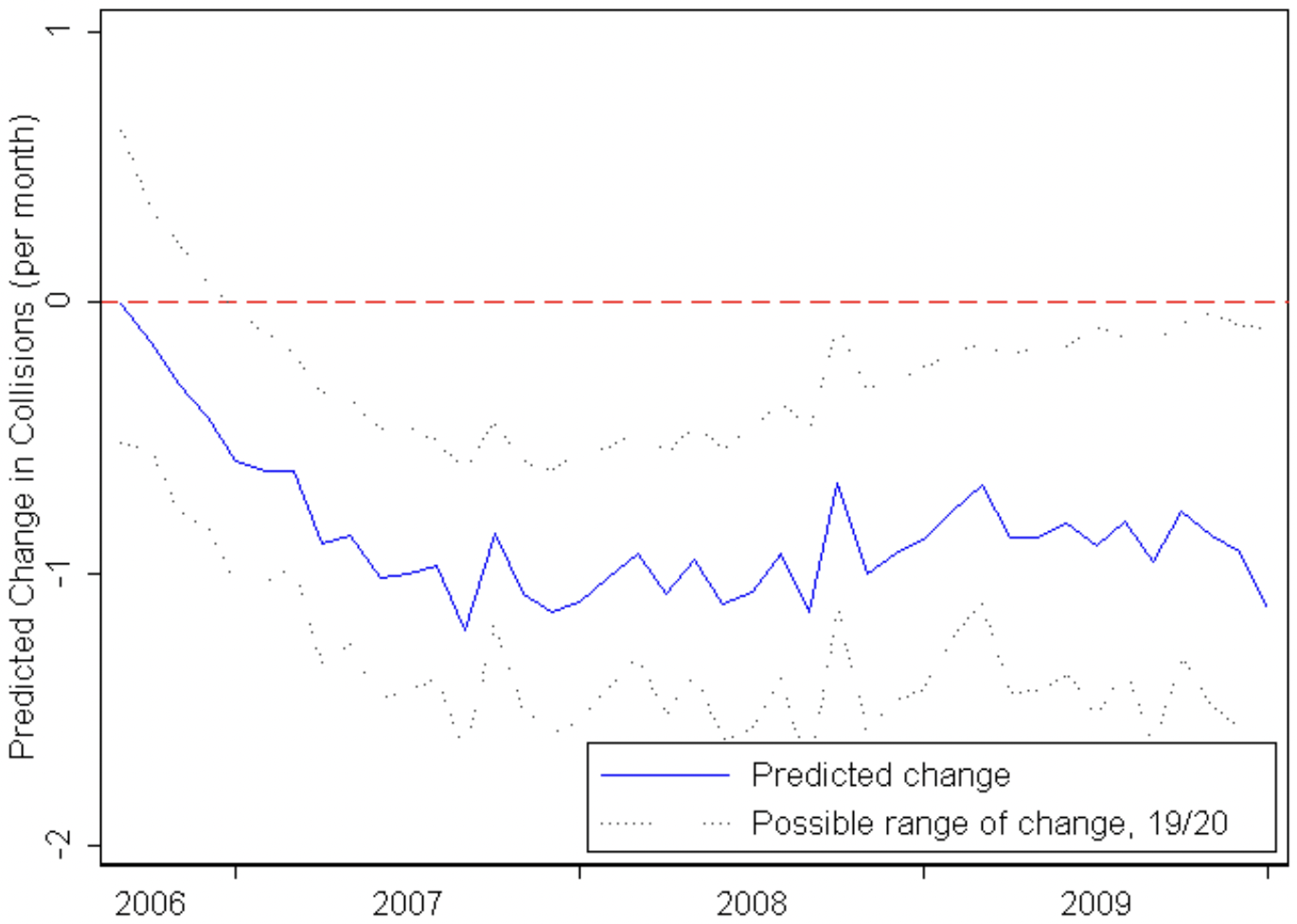
In each figure, the thick blue line tracks the predicted reduction in collisions at an approach that gets a camera. The black dotted lines are the high and low boundaries of a 95 percent confidence range. Since these are simulated values, this range tracks how sure we can be about the predictions. The dashed red line denotes zero change from the baseline. When all three lines are below the dashed red line, the change in collisions is statistically significant and we can be confident that the presence of the camera reduces collisions.
The Effect of One Camera
Figure 3 — Predicted Reduction in Collisions per Month; Camera Installed on a Different Approach in 09/2006
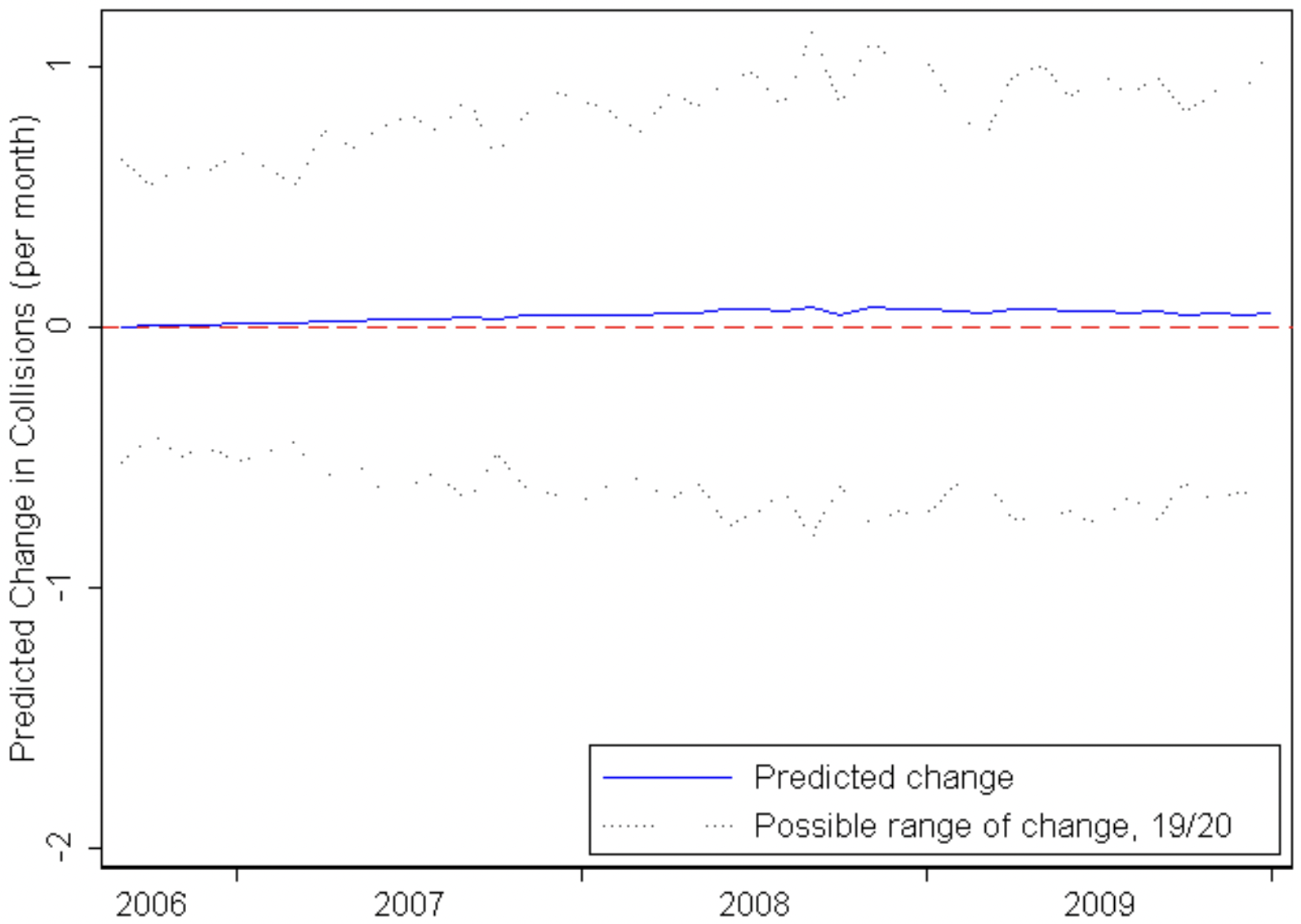
Within 12 months of installing one camera at a high-risk intersection, we estimate there will be about one less collision every month and this drop will be permanent. This can be seen in Figure 2.
The data indicates that the effect of one camera on the other three approaches in the intersection is virtually nil. In Figure 3 you can see the predicted number of collisions at a simulated intersection approach that never receives a camera, but which shares an intersection with one approach that does have a camera. There is no apparent spillover effect from one camera.
The Effect of Two Cameras
Figure 4 — Predicted Reduction in Collisions per Month; Camera on Different Approach in 09/2006 Than on This Approach in 08/2007
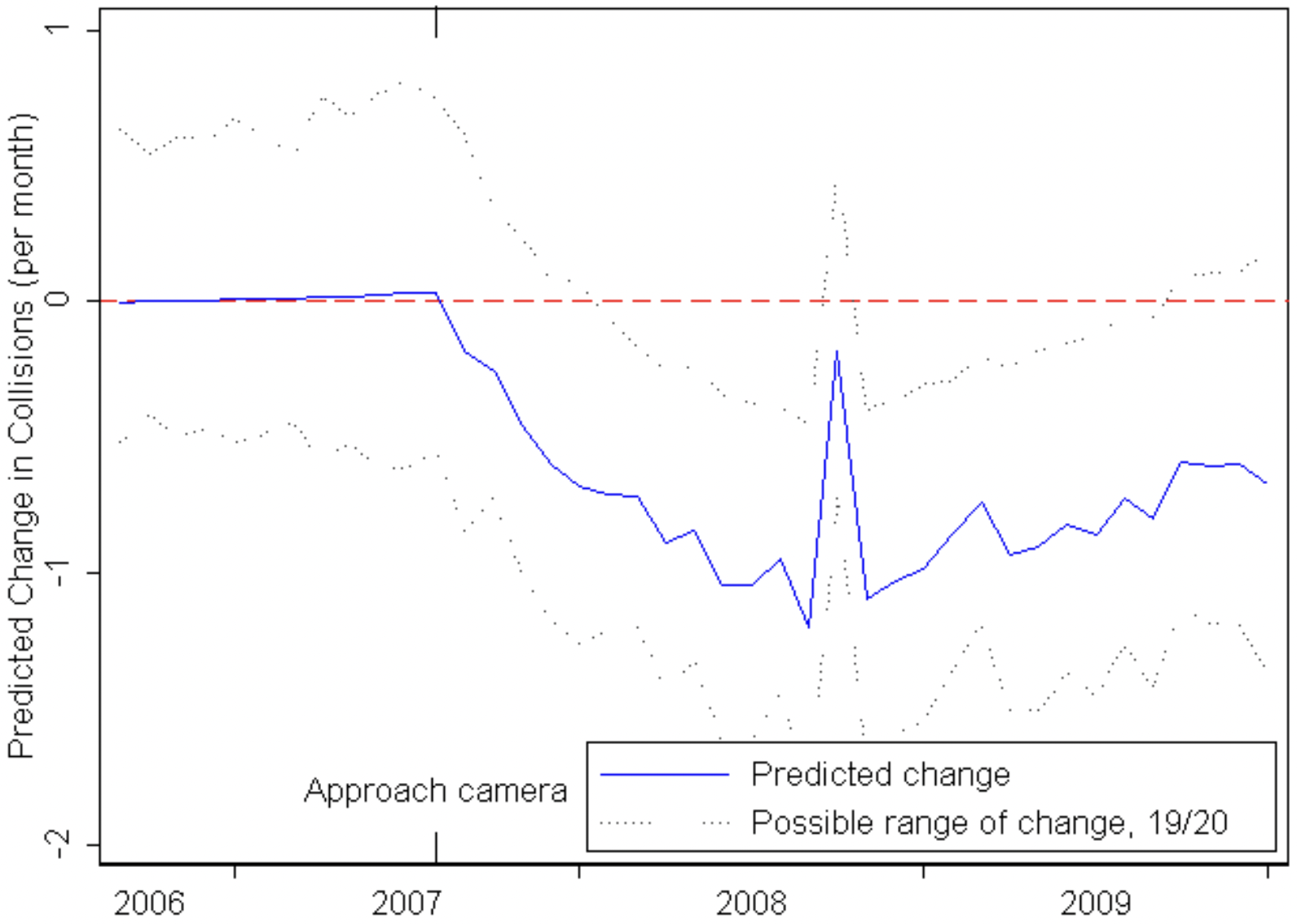
Our data suggests that two cameras at the same intersection bring down the number of collisions substantially more than one camera alone. Figure 4 shows our prediction for an approach that does not receive a camera at first, but then later has a camera installed. The first camera, again, has almost no impact on the approach without a camera.
Table 2 — Estimated Average Reduction in Monthly Collisions for High-risk Intersections*
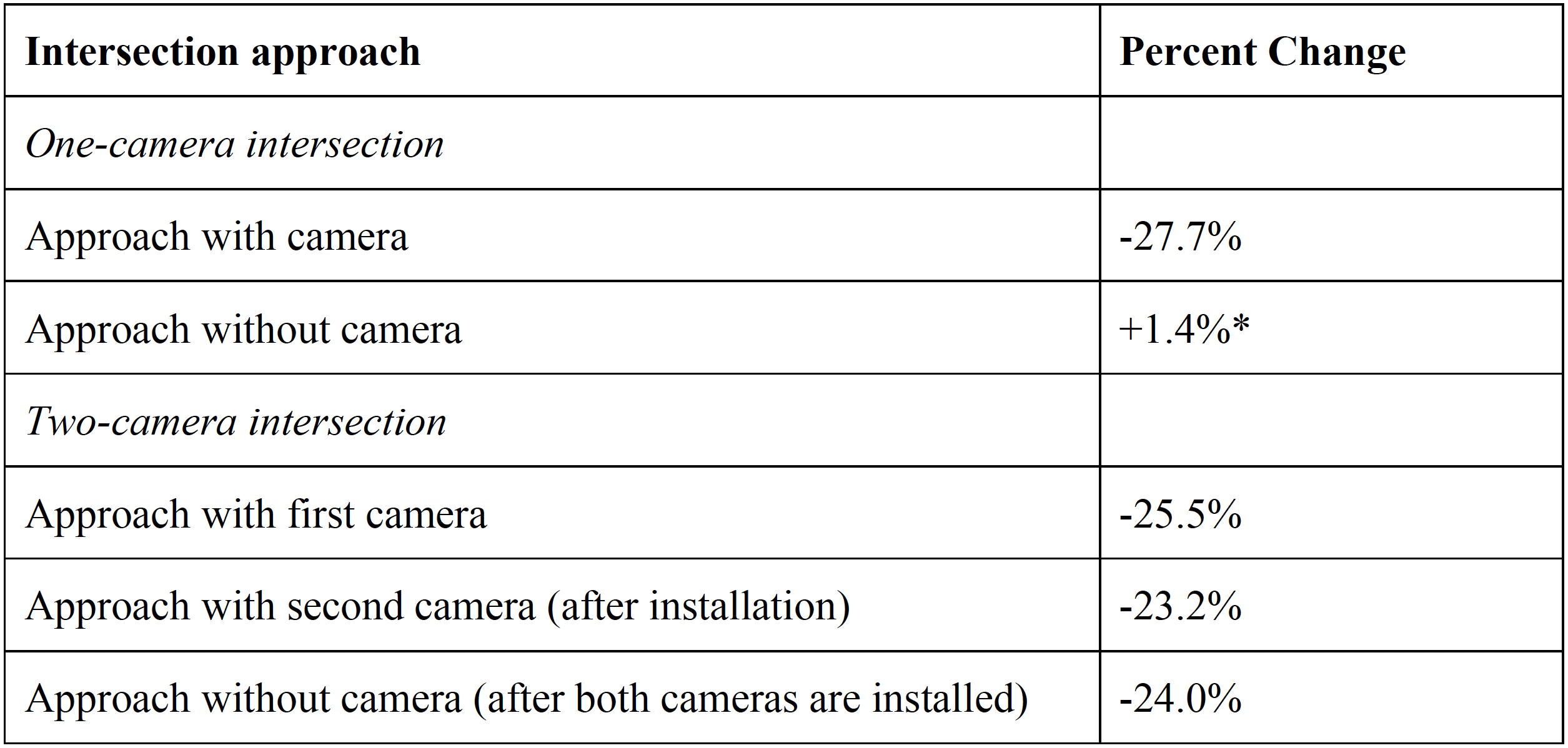
Figure 5 — Predicted Reduction in Collisions per Month; Camera on Two Other Approaches Installed in 09/2006 and in 08/2007
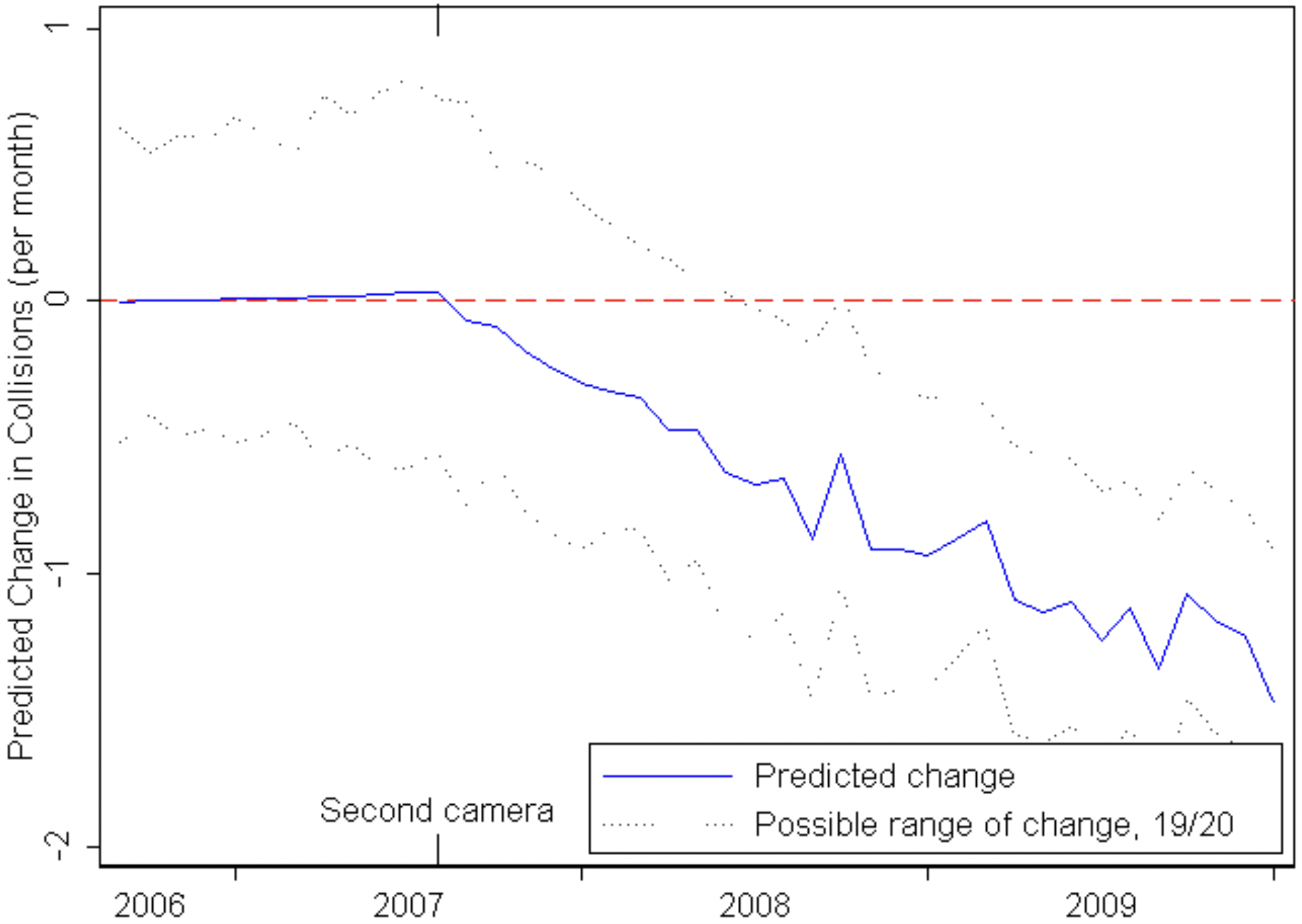
The effects are basically a combination of the previous two figures. After the first camera is installed, we see no change in the approach without a camera. However, once the approach we simulate gets a camera there is a clear reduction in accidents. With two cameras in the intersection, there is also a strong spillover reduction in collisions, even on the two approaches without any camera, as you see in Figure 5.
Table 2 lists the expected reduction in collisions compared to the baseline for various situations.
A Real Life Example of Spillover Effects: FM 1960 West at Tomball Parkway
Figure 6 — Actual Collisions at FM 1960 at Tomball Parkway 2003–2009; Cameras Installed November 2006 and August 2007

Consider the example of FM 1960 West at Tomball Parkway. As Table 3 shows, this intersection was the most prone to collisions in Houston before cameras were installed. There was more than a 50 percent reduction in collisions after cameras were installed. We used our model to simulate what would have happened without cameras at this intersection. Simulations suggest that these reductions were primarily due to the spillover effect from this intersection receiving two cameras.
Figure 7 — Predicted Increase in Collisions Compared to Two Cameras; FM 1960 W at Tomball Pkwy
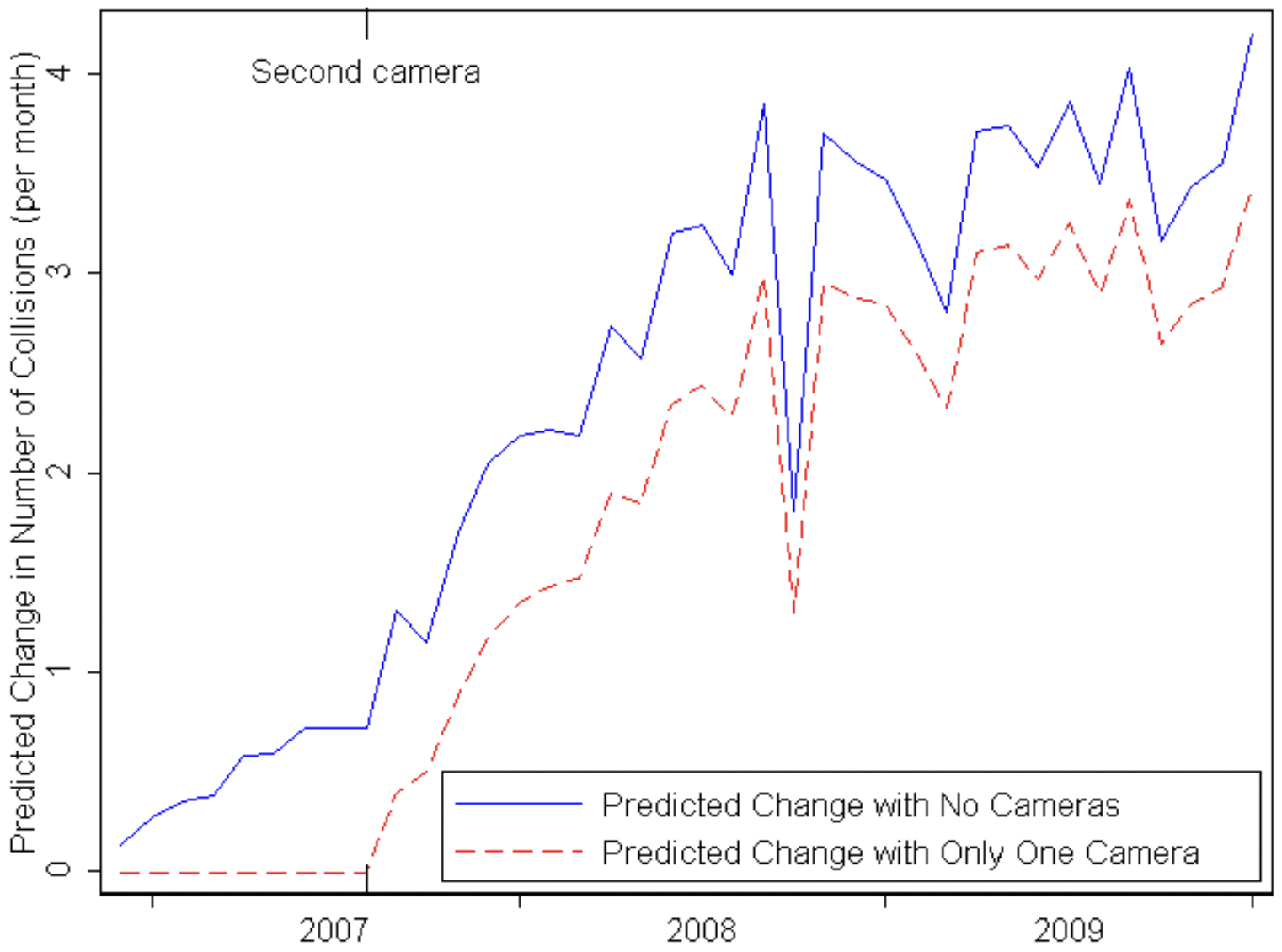
Figure 6 shows the actual timeline of monthly collisions at the intersection. In Figure 7, we predict the number of collisions would have been much larger with no cameras or only one camera at the intersection in the same period.
Conclusion
In Houston, intersection approaches with cameras experienced 1,413 collisions between September 2006 and December 2009. Our findings suggest that this is not a fluke. Simulated recreations of this period estimate that we should expect about 1,4389 collisions at these approaches with cameras. If we simulate what this number would have been without cameras installed, we would expect about 2,23010—an increase of almost 800 collisions.
The evidence suggests that even when we account for other factors that contribute to traffic accidents, cameras appear to reduce the number of wrecks. An approach with a camera for the full three years of our data tended to have about 28 percent fewer collisions than the baseline expectation, and intersections with more than one camera saw stronger benefits once the second camera was installed.
The most surprising trend we uncovered is that intersections with one camera only benefit from reductions in accidents at the approach with the camera. Adding a second camera, however, brings reductions in the number of collisions not only on the newly monitored approach but also on the other two approaches without cameras.
Endnotes
1. We gratefully acknowledge the assistance of the Houston-Galveston Area Council of Governments and Jeff Kaufman for their assistance in providing us with access to the CRIS database.
2. Texas Transportation Code, Section 550.061.
3. DARLEP is an acronym for Digital Automated Red Light Enforcement Program.
4. See http://www.txdot.gov/driversvehicles/crashrecords/reports.htm.
5. Eighteen intersections received one additional camera and one intersection received two additional cameras.
6. This is an average of .84 collisions per month at approaches that eventually received cameras, compared to .68 collisions per month on average at approaches that never got a camera. The difference is large and statistically significant at the .05 level, meaning that if there really is no difference between the two groups then a difference this large is so rare it would happen fewer than five times out of 100.
7. In statistical terms, we employ fixed effects by intersection approach and calendar month.
8. We employ a negative binomial regression with the control variables described above and fixed effects by intersection, approach, direction and month. Each of the 84 individual predicted monthly collision counts is calculated from 1,000 sets of simulated model parameters.
9. The 95 percent confidence interval for this estimate is between 1,061 and 1,913.
10. The 95 percent confidence interval for this estimate is between 1,518 and 3,167.
Appendix
Table 3 — Full List of Intersections and Actual Average Monthly Collisions Before and After Camera Installation; Not Controlled for Number of Cameras or Timing of Installation
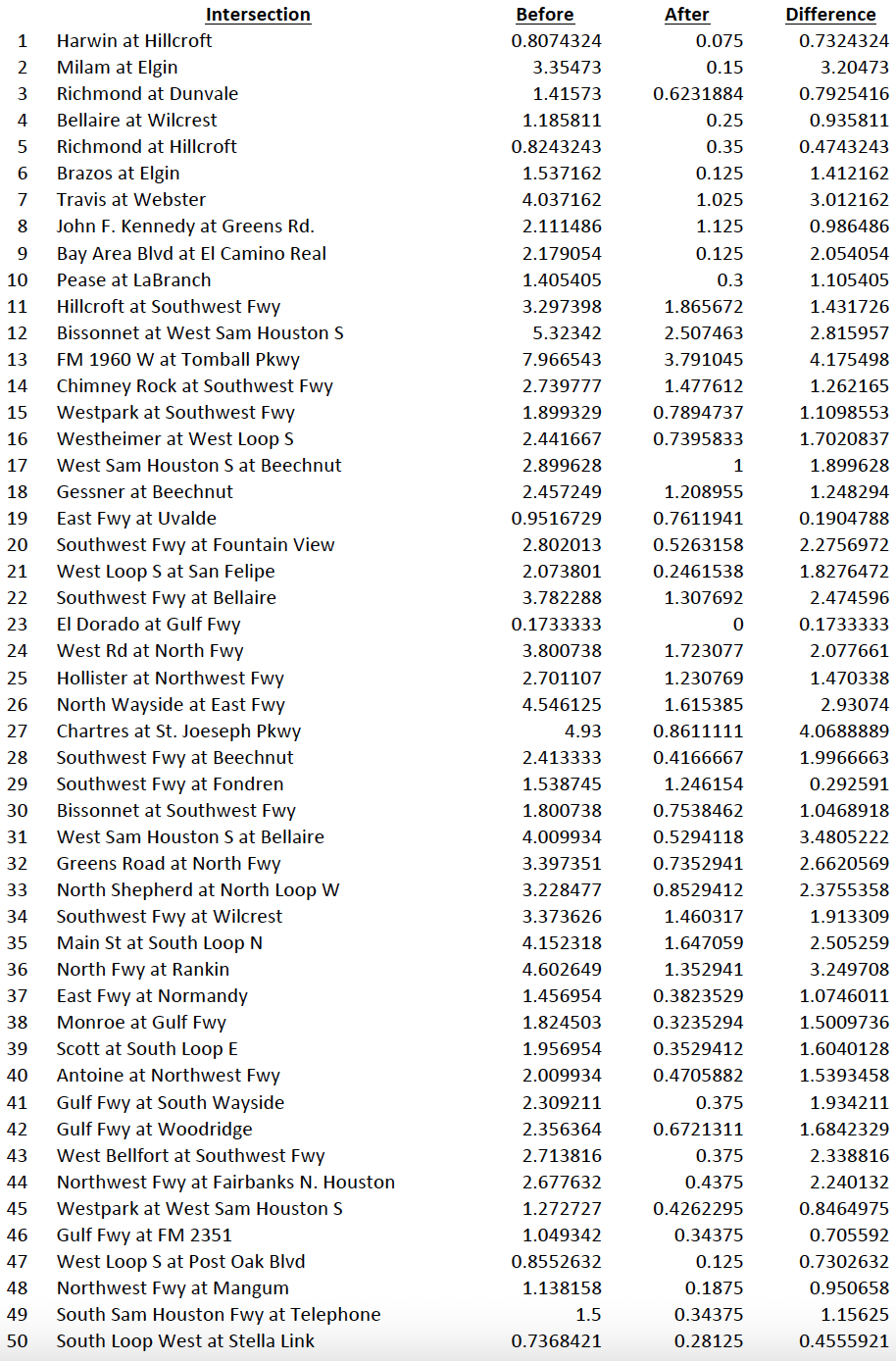
This material may be quoted or reproduced without prior permission, provided appropriate credit is given to the author and Rice University’s Baker Institute for Public Policy. The views expressed herein are those of the individual author(s), and do not necessarily represent the views of Rice University’s Baker Institute for Public Policy.